Performing Melanoma Diagnosis by an Effective Multi-view Convolutional Network Architecture
Article Ecrit par: Reyes, Oscar ; Pérez, Eduardo ;
Résumé: Despite the proved effectiveness of deep learning models in solving complex problems, the melanoma diagnosis remains as a challenging task mainly due to the high levels of inter and intra-class variability present in images of moles. Aimed at filling this gap, in this work we propose a novel architecture for melanoma diagnosis which is inspired on multi-view learning and data augmentation. In order to make the model more transformation-invariant, the proposed architecture creates different independent and specific views of an image. The transformations applied on the original images are learned by genetic algorithms which find the best set of transformations. Also, the final predictions are yielded by aggregating information that comes from independent views. To evaluate the suitability of the proposal, an extensive experimental study on fifteen public melanoma-image datasets was conducted. The impact of the parameters of the genetic algorithms on the proposed architecture were analyzed, and it was also demonstrated that the proposed architecture attained better results when using transformations derived from the genetic algorithm than using random transformations. Finally, the results showed the suitability of the proposed model, where four state-of-the-art data augmentation techniques were significantly outperformed.
Langue:
Anglais
Thème
Informatique
Mots clés:
Genetic algorithms
Melanoma diagnosis
Convolutional neural networks (CNNs)
Data augmentation
Multi-view learning
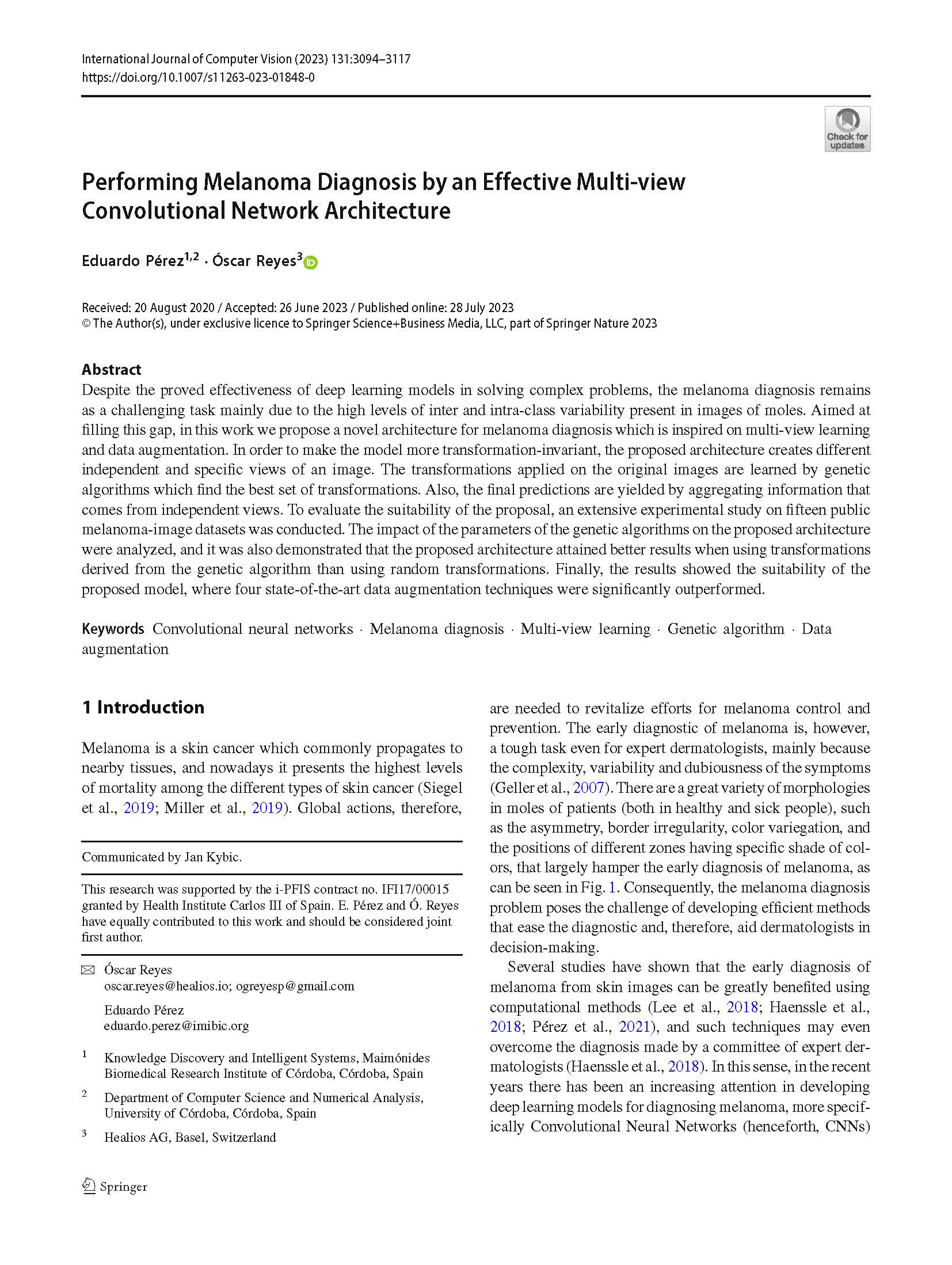