Improving collaborative recommendation based on item weight link prediction
Article Ecrit par: Dahmani, Youcef ; Nouali, Omar ; Kharroubi, Sahraoui ;
Résumé: There is a continuous information overload on the Web. The problem treated is how to have relevant items (documents, products, services, etc.) at time and without difficulty. Filtering system also called recommender systems are widely used to recommend items to users by similarity process such as Amazon, MovieLens, Cdnow, etc. In the literature, to predict a link in a bipartite network, most methods are based either on a binary history (like, dislike) or on the common neighbourhood of the active user. In this paper, we modelled the recommender system by a weighted bipartite network. The bipartite topology offers a bidirectional reasoning item side and user side, which preserve the information shared between the nodes. To make such a prediction, we seek to determine the shares of items shared between users. In the first step, we accumulate the shares of the users towards the items and in the second step, the shares of the items towards the users. The idea is to exploit the item-user connectivity to predict nonexistent links based on existing links. Therefore, the information is propagated linearly and without loss between nodes in the network. We focused on the item through a dual projection that combines the sharing of quotas between users and items. Empirical tests on real data sets (Amazon-Books, MovieLens 1M, Yelp2018, Yahoo! Songs) showed satisfactory results.
Langue:
Anglais
Thème
Informatique
Mots clés:
Ranking
Recommender systems
Information propagation
Item-user
weighted network
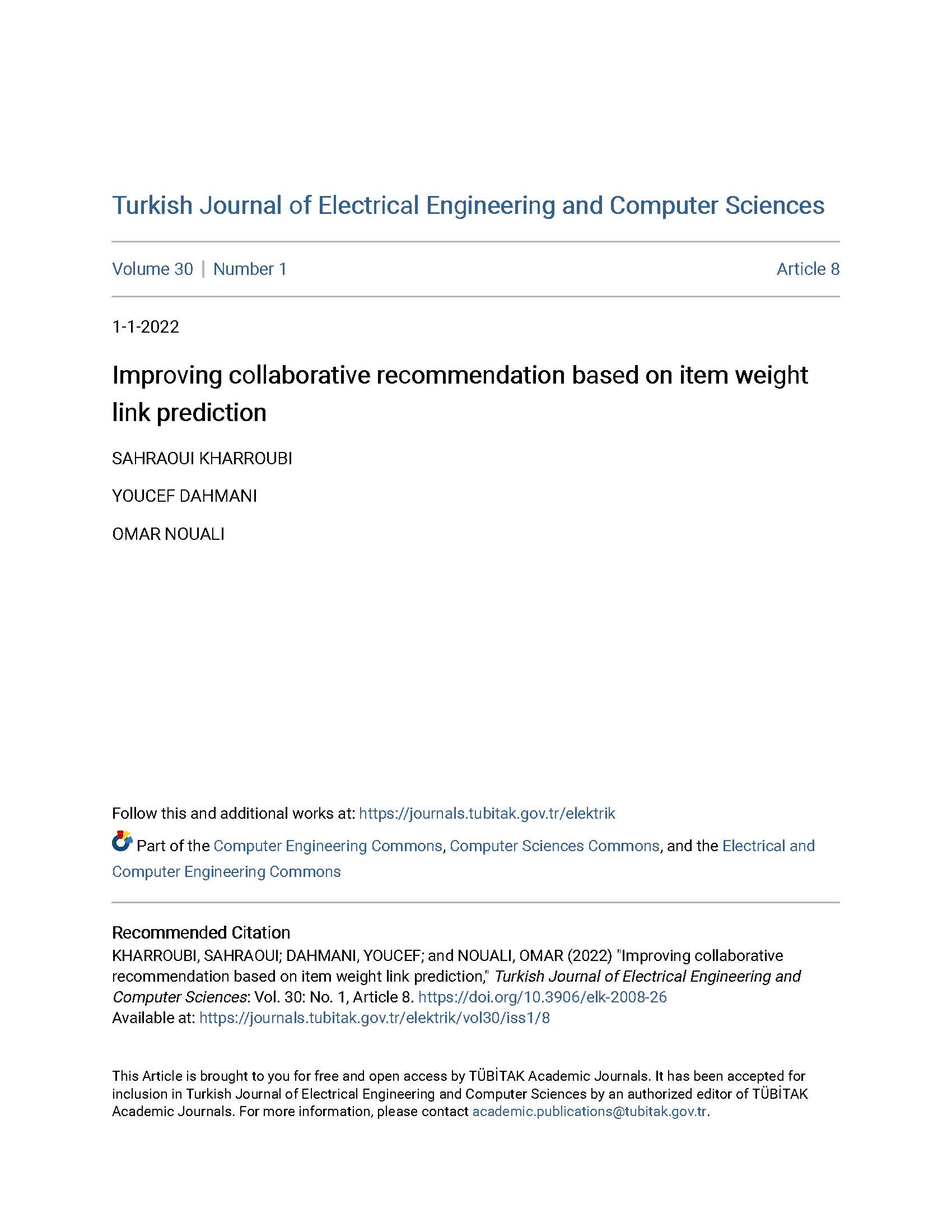