Survey of Machine Learning based intrusion detection methods for Internet of Medical Things
Article Ecrit par: Al-Garadi, Mohammed Ali ; Boustia, Narhimene ; Si-Ahmed, Ayoub ;
Résumé: The Internet of Medical Things (IoMT) has revolutionized the healthcare industry by enabling physiological data collection using sensors, which are transmitted to remote servers for continuous analysis by physicians and healthcare professionals. This technology offers numerous benefits, including early disease detection and automatic medication for patients with chronic illnesses. However, IoMT technology also presents significant security risks, such as violating patient privacy or exposing sensitive data to interception attacks due to wireless communication, which could be fatal for the patient. Additionally, traditional security measures, such as cryptography, are challenging to implement in medical equipment due to the heterogeneous communication and their limited computation, storage, and energy capacity. These protection methods are also ineffective against new and zero-day attacks. It is essential to adopt robust security measures to ensure data integrity, confidentiality, and availability during data collection, transmission, storage, and processing. In this context, using Intrusion Detection Systems (IDS) based on Machine Learning (ML) can bring a complementary security solution adapted to the unique characteristics of IoMT systems. Therefore, this paper investigates how IDS based on ML can address security and privacy issues in IoMT systems. First, the generic three-layer architecture of IoMT is provided, and the security requirements of IoMT systems are outlined. Then, the various threats that can affect IoMT security are identified, and the advantages, disadvantages, methods, and datasets used in each solution based on ML at the three layers that make up IoMT are presented. Finally, the paper discusses the challenges and limitations of applying IDS based on ML at each layer of IoMT, which can serve as a future research direction.
Langue:
Anglais
Thème
Informatique
Mots clés:
Privacy
security
Machine learning
Internet of Medical Things
Intrusion Detection System
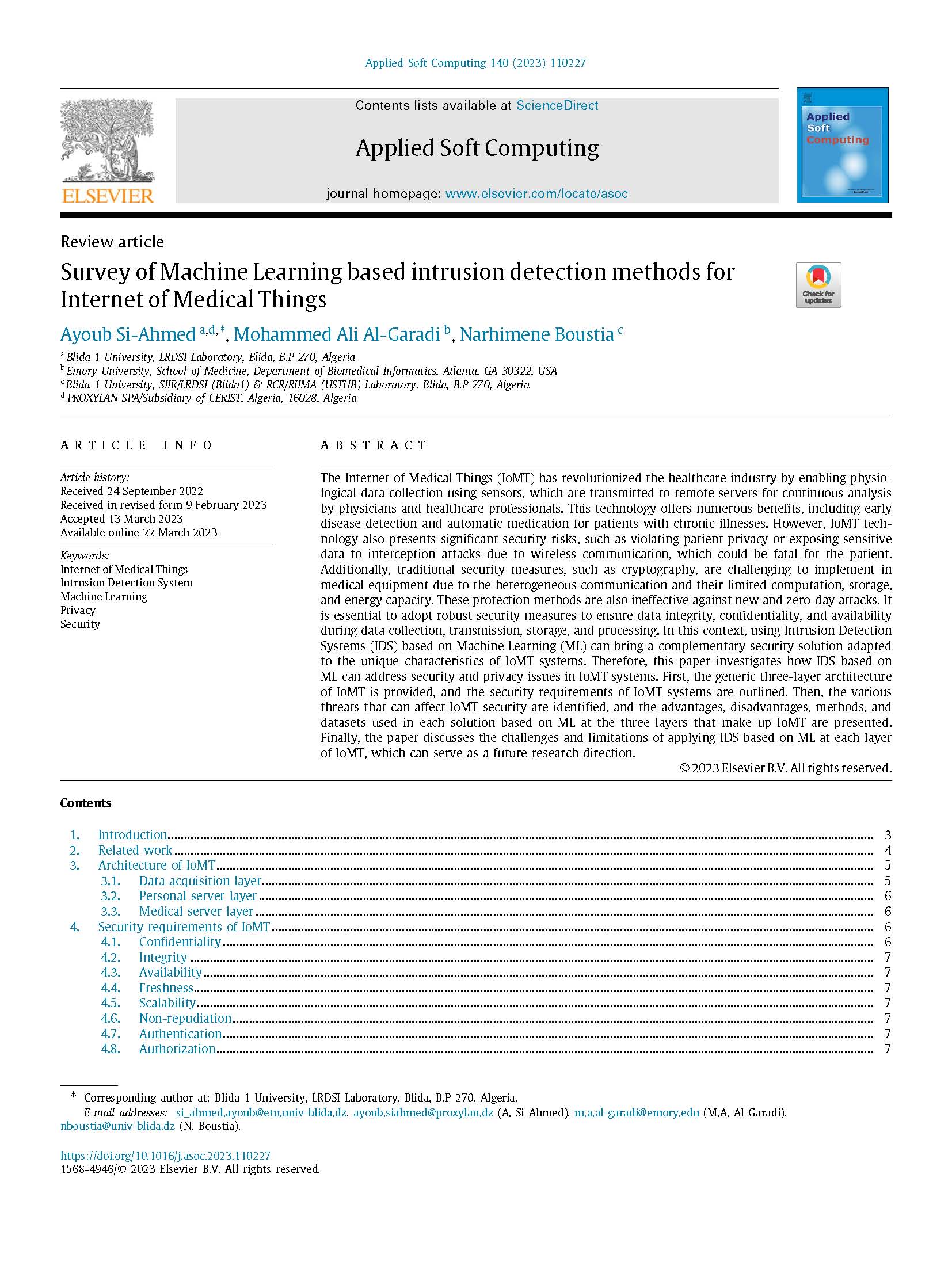