Energy load forecasting
one-step ahead hybrid model utilizing ensembling
Article Ecrit par: Koukaras, Paraskevas ; Tjortjis, Christos ; Mystakidis, Aristeidis ; Ioannidis, Dimosthenis ; Tsalikidis, Nikos ;
Résumé: In the light of the adverse effects of climate change, data analysis and Machine Learning (ML) techniques can provide accurate forecasts, which enable efficient scheduling and operation of energy usage. Especially in the built environment, Energy Load Forecasting (ELF) enables Distribution System Operators or Aggregators to accurately predict the energy demand and generation trade-offs. This paper focuses on developing and comparing predictive algorithms based on historical data from a near Zero Energy Building. This involves energy load, as well as temperature data, which are used to develop and evaluate various base ML algorithms and methodologies, including Artificial Neural Networks and Decision-trees, as well as their combination. Each algorithm is fine-tuned and tested, accounting for the unique data characteristics, such as the presence of photovoltaics, in order to produce a robust approach for One-Step-Ahead ELF. To this end, a novel hybrid model utilizing ensemble methods was developed. It combines multiple base ML algorithms the outputs of which are utilized to train a meta-model voting regressor. This hybrid model acts as a normalizer for any new data input. An experimental comparison of the model against unseen data and other ensemble approaches, showed promising forecasting results (mean absolute percentage error = 5.39%), particularly compared to the base algorithms.
Langue:
Anglais
Thème
Informatique
Mots clés:
Machine learning
Time series forecasting
Ensemble methods
Smart buildings
Energy load forecasting
Short-term prediction
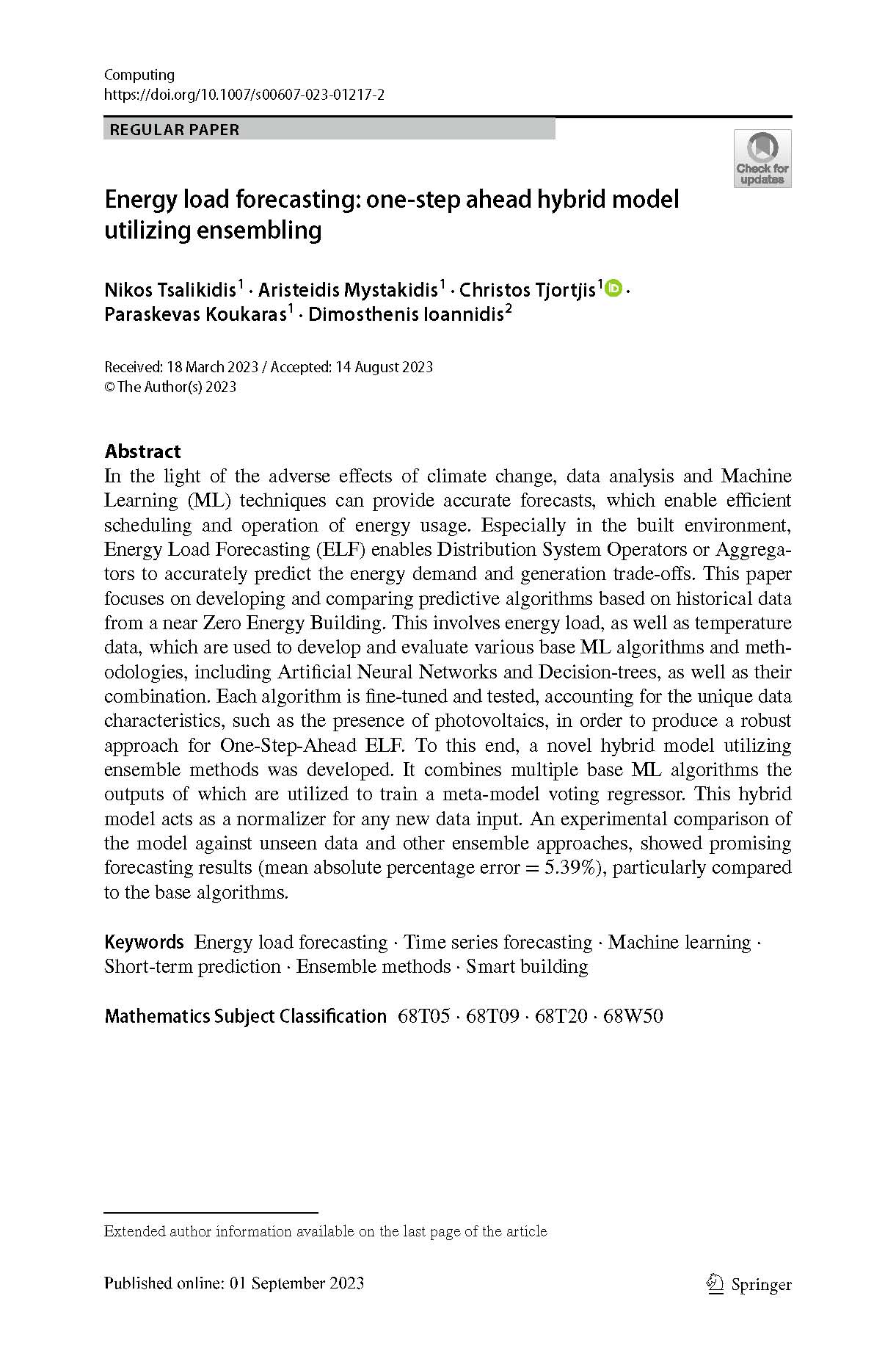