Machine learning using context vectors for object coreference resolution
Article Ecrit par: Thanh, Tho Quan ; Phan Thi, Tuoi ; Le Thi, Thuy ;
Résumé: Object coreference resolution is used in sentiment analysis to identify sentiment words referring to an aspect of an object in a document. However, this poses a challenge in natural language processing and is consequently an area of ongoing research. Further, to the best of our knowledge, object coreference resolution with more than one object has not been given much attention. To effectively address object coreference resolution, this paper proposes a method in which machine learning is applied to a large volume of textual data represented by context vectors, constituting a new form of language representation. The proposed machine learning model uses these vectors to achieve state-of-the-art performance in object coreference resolution. In addition, a combination of dependency grammar, sentiment ontology, and coreference graphs is used to obtain triplets of object, aspect, and sentiment. In experiments conducted on sentiment textual data obtained from Amazon.com, the proposed method achieved an average coreference resolution of object, aspect, and sentiment precision value of approximately 90%. This result suggests that the proposed method can contribute considerably to the field of object coreference resolution, and further research is therefore warranted.
Langue:
Anglais
Index décimal
006.3 .Intelligence artificielle (ouvrages généraux sur l'intelligence artificielle et la science cognitive, reconnaissance de formes comme outil de l'intelligence artificielle, systèmes de questions et réponses)
Thème
Informatique
Mots clés:
sentiment analysis
Object coreference resolution
Object aspect
Sentiment ontology
CROAS
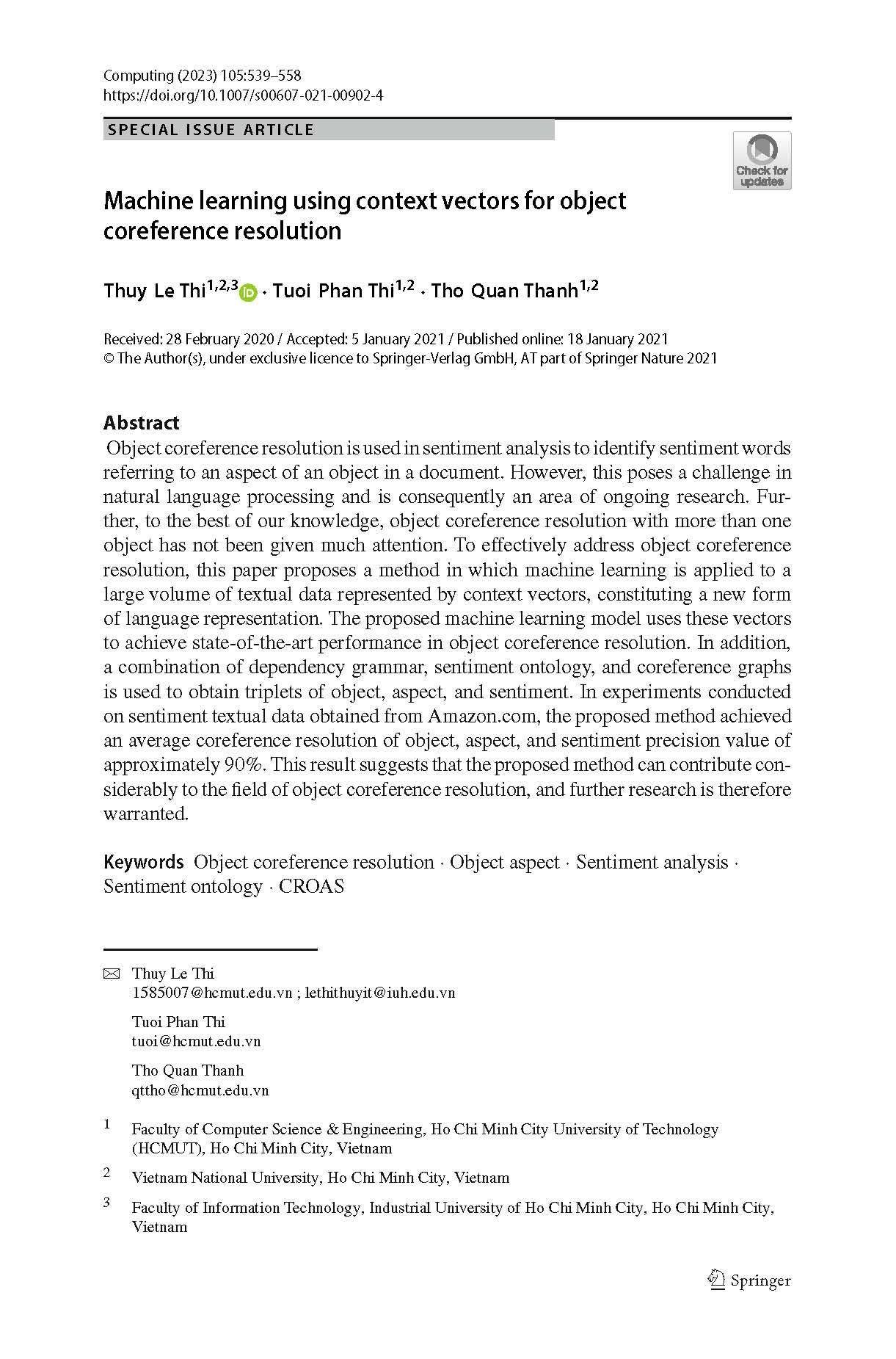