Multi-level granularity entropies for fuzzy coverings and feature subset selection
Article Ecrit par: Li, Jinjin ; Huang, Zhehuang ;
Résumé: Various fuzzy covering based rough set models can characterize and approximate a given target concept with different lower and upper approximation operators. However, due to the lack of effective construction method and semantic interpretation on real data, there are few investigation on a comprehensive uncertain measure for fuzzy covering, as well as its applications in feature subset selection. For this reason, a new uncertain measure called multi-level granularity entropy is proposed by means of different fuzzy neighbourhoods. First, five types of granularity structure and granularity entropy for fuzzy covering are studied; Second, a multi-level granularity entropy is introduced, using which one can evaluate the discrimination information implied in a family of fuzzy coverings from different hierarchical levels. Some variants of the multi-level granularity entropy, such as conditional granularity entropy, joint granularity entropy, and mutual granularity entropy, are then proposed to reflect the change of uncertain information. Finally, the dimensionality reduction is implemented in the light of maintaining the discrimination ability, and a forward feature selection algorithm is developed by using the multi-level entropy. Extensive numerical experiments demonstrate that the presented model exhibits better performance in feature learning, and outperforms some representative feature selection algorithms in terms of classification accuracy and the number of selected features.
Langue:
Anglais
Thème
Informatique
Mots clés:
Feature selection
Rough sets
Granularity entropy
Fuzzy covering
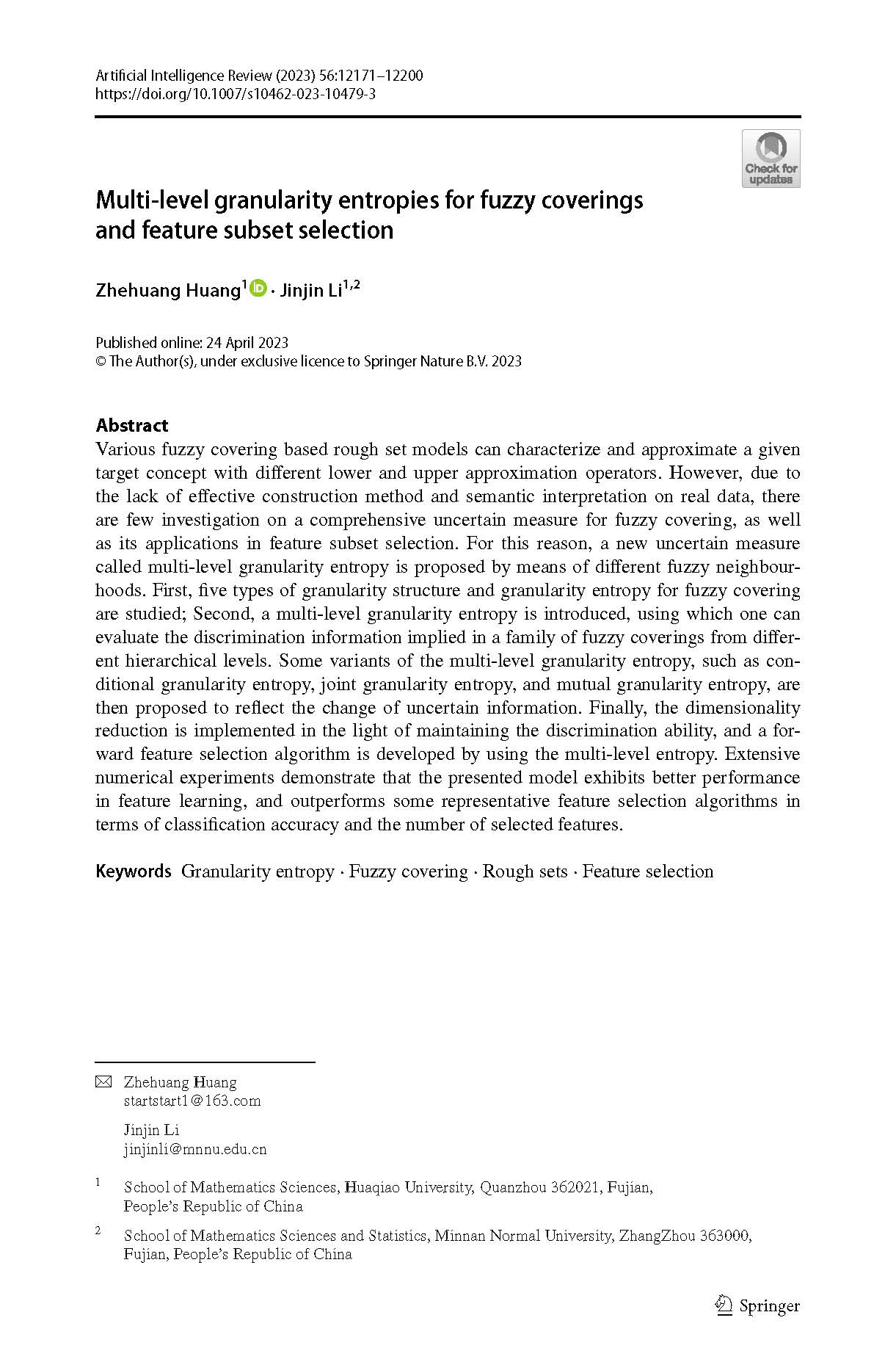