An extensive survey on the use of supervised machine learning techniques in the past two decades for prediction of drug side effects
Article Ecrit par: Mazumder, Dilwar Hussain ; Das, Pranab ;
Résumé: Approved drugs for sale must be effective and safe, implying that the drug's advantages outweigh its known harmful side effects. Side effects (SE) of drugs are one of the common reasons for drug failure that may halt the whole drug discovery pipeline. The side effects might vary from minor concerns like a runny nose to potentially life-threatening issues like liver damage, heart attack, and death. Therefore, predicting the side effects of the drug is vital in drug development, discovery, and design. Supervised machine learning-based side effects prediction task has recently received much attention since it reduces time, chemical waste, design complexity, risk of failure, and cost. The advancement of supervised learning approaches for predicting side effects have emerged as essential computational tools. Supervised machine learning technique provides early information on drug side effects to develop an effective drug based on drug properties. Still, there are several challenges to predicting drug side effects. Thus, a near-exhaustive survey is carried out in this paper on the use of supervised machine learning approaches employed in drug side effects prediction tasks in the past two decades. In addition, this paper also summarized the drug descriptor required for the side effects prediction task, commonly utilized drug properties sources, computational models, and their performances. Finally, the research gap, open problems, and challenges for the further supervised learning-based side effects prediction task have been discussed.
Langue:
Anglais
Thème
Informatique
Mots clés:
Deep learning
Machine learning
Supervised learning
Drug side effects
Drug properties
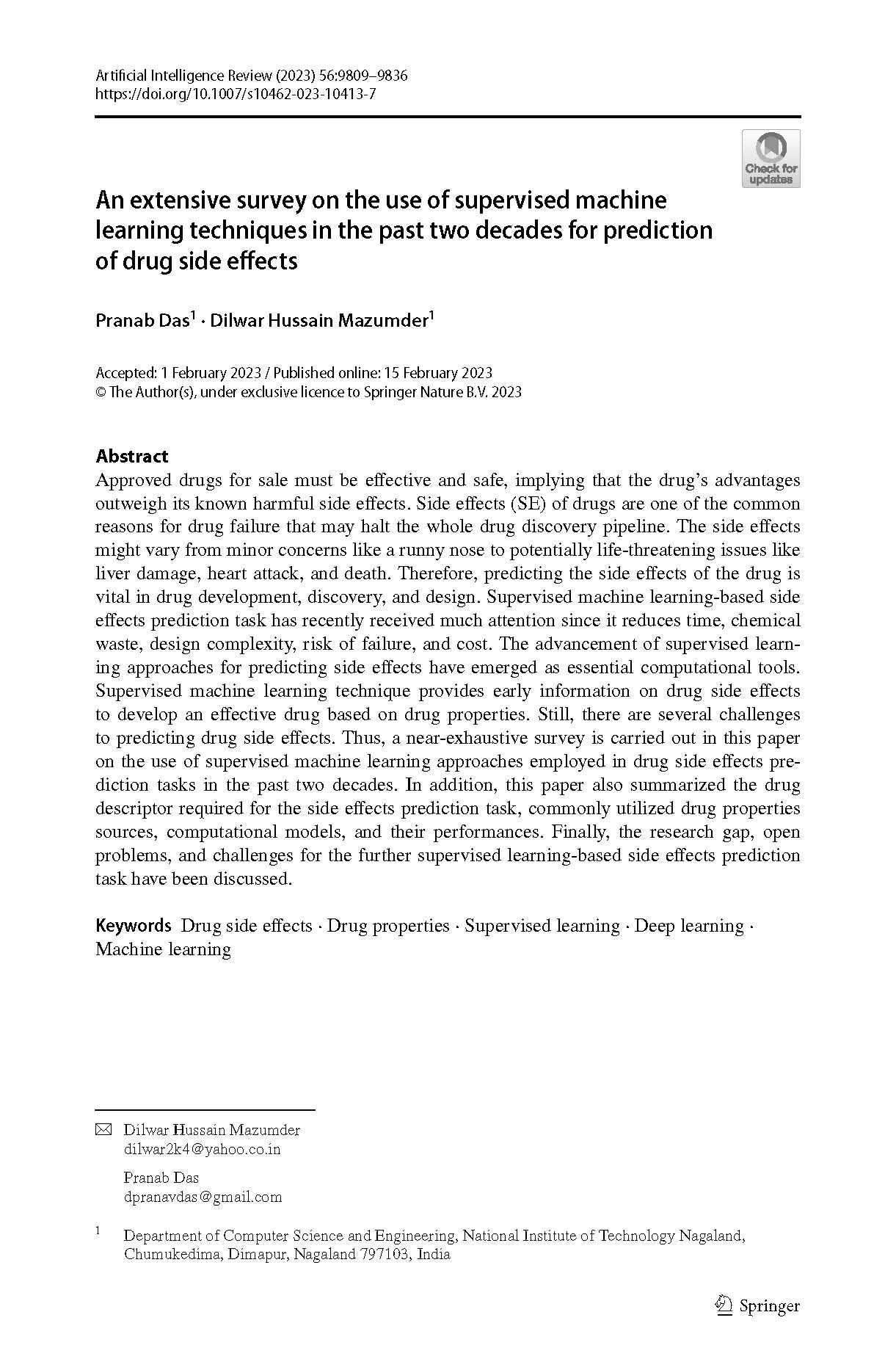