Probing the Impacts of Visual Context in Multimodal Entity Alignment
Article Ecrit par: Zhang, Ziheng ; Yang, Han ; Shi, Yinghui ; Lin, Zhenxi ; Zheng, Yefeng ; Wang, Meng ;
Résumé: We study the problem of multimodal embedding-based entity alignment (EA) between different knowledge graphs. Recent works have attempted to incorporate images (visual context) to address EA in a multimodal view. While the benefits of multimodal information have been observed, its negative impacts are non-negligible as injecting images without constraints brings much noise. It also remains unknown under what circumstances or to what extent visual context is truly helpful to the task. In this work, we propose to learn entity representations from graph structures and visual context, and combine feature similarities to find alignments at the output level. On top of this, we explore a mechanism which utilizes classification techniques and entity types to remove potentially un-helpful images (visual noises) during alignment learning and inference. We conduct extensive experiments to examine this mechanism and provide thorough analysis about impacts of the visual modality on EA.
Langue:
Anglais
Thème
Informatique
Mots clés:
Multimodality
knowledge graphs
Entity alignment
Visual context
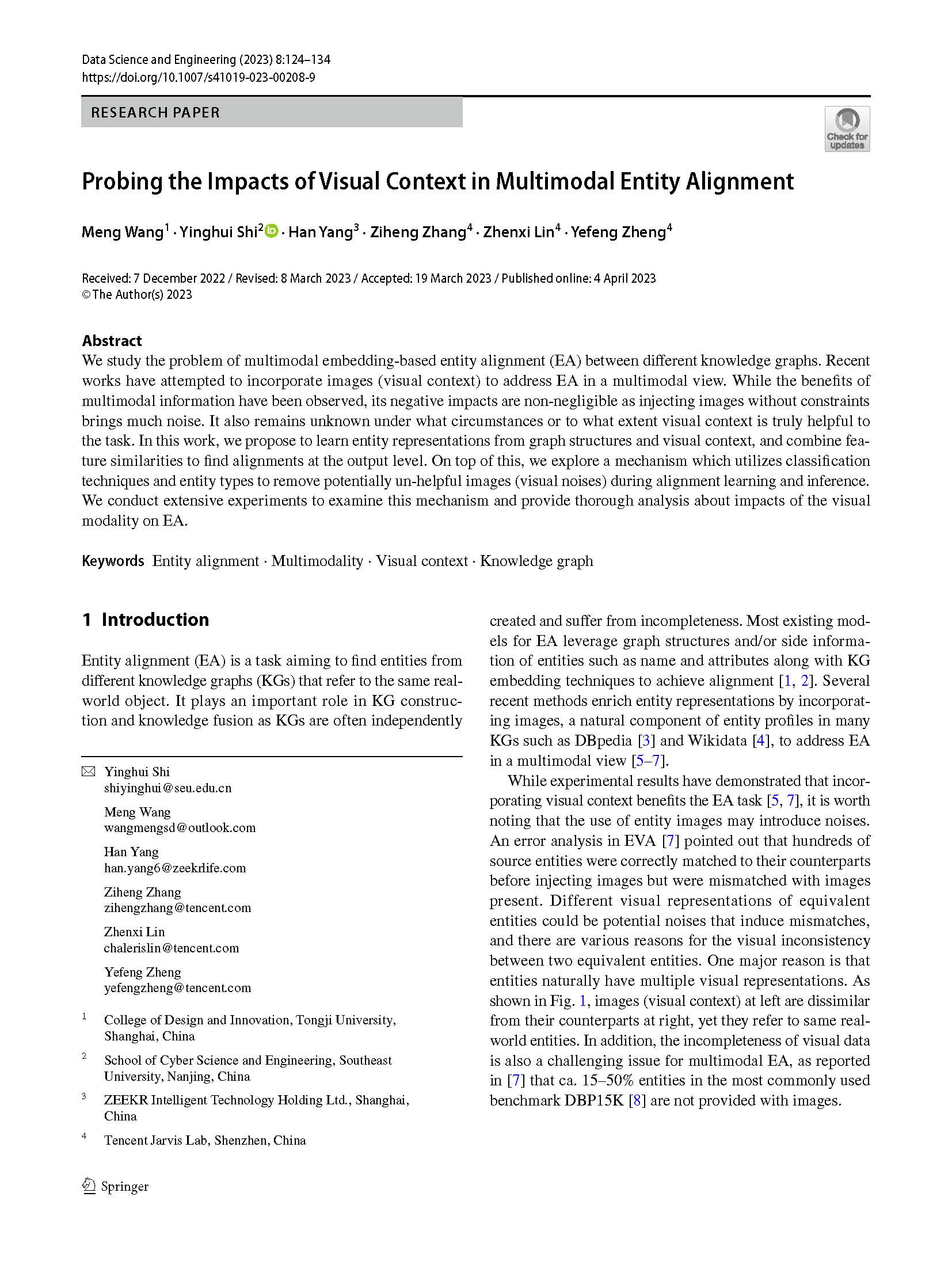