A cloud computing load prediction hybrid model with adaptive weight strategy
Article Ecrit par: Guan, Yepeng ; Xiong, Chao ;
Résumé: In recent years, cloud computing has revolutionized computing. The resource usage prediction of machine workloads has been one of the most important and challenging problems. Load prediction in cloud computing is challenging due to the inefficiency of feature extraction and complex loading environment. Attention mechanism based Multi-level Feature Extraction (MFE) model has been developed. Empirical mode decomposition has been selected to extract the sequence surface features. Multi-level LSTM has been used to extract multi-level features of the decomposed load in the empirical mode decomposition. Attention mechanism has been adopted to get different weights for the extracted features. In order to further get causal relationship of time series data, Empirical mode decomposition based Temporal Convolutional Network (ETCN) model has been developed. Multiple temporal convolutional network residual blocks are stacked to improve the historical memory ability and increase the local receptive field. An adaptive weight strategy has been proposed to assign different weights to the developed MFE and ETCN. The weights are updated adaptively according to the errors in MFE and ETCN, respectively. The proposed method has excellent performance in cloud computing on some challenging datasets by comparison with some state-of-the-art ones. The proposed method achieves an average improvement of 30.23% and 41.5% on the NASA datasets and Saskatchewan datasets, respectively.
Langue:
Anglais
Thème
Informatique
Mots clés:
Cloud computing load prediction
Multi-level feature extraction model
Adaptive weight update
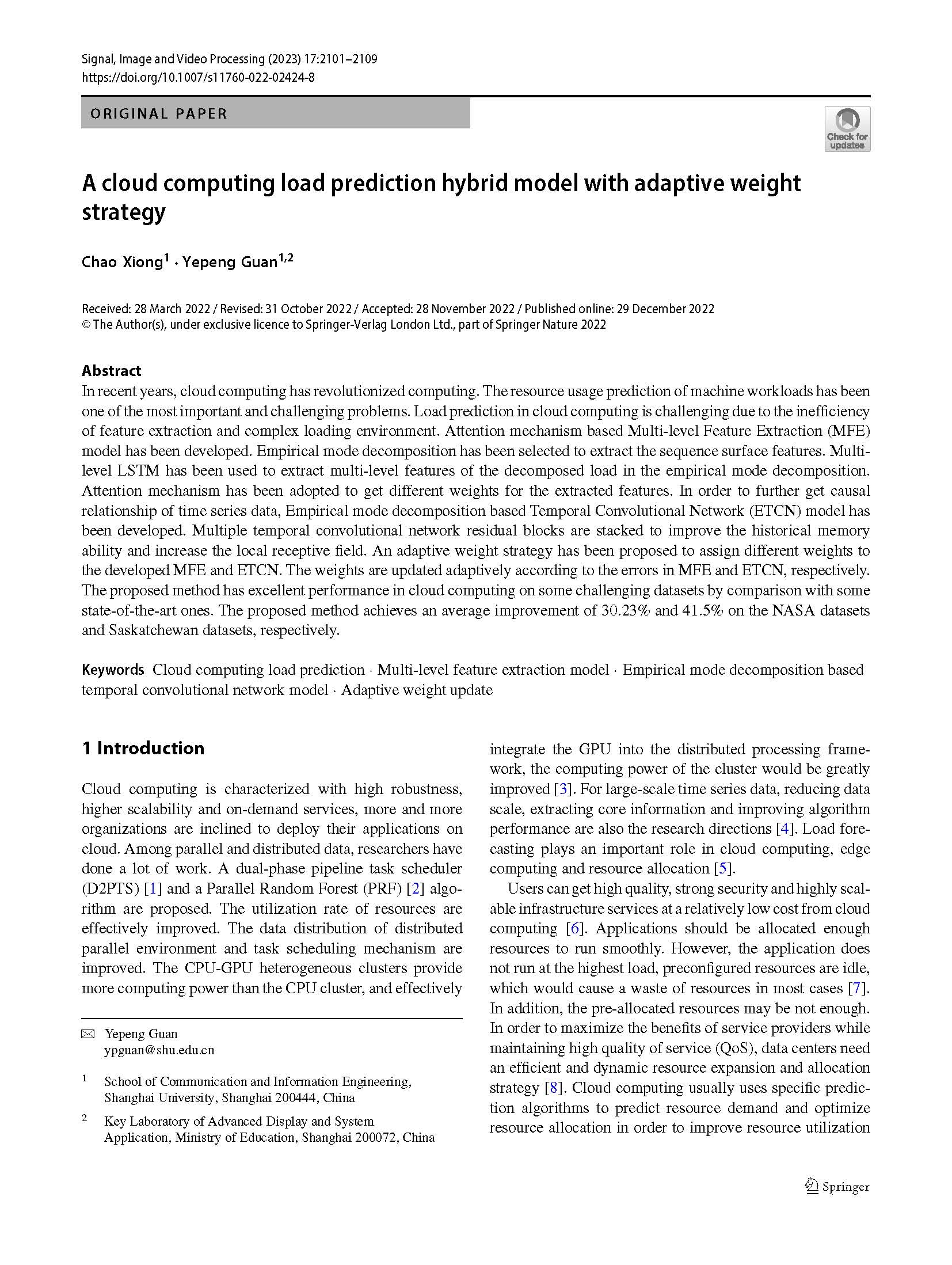