Generalized hurdle count data models based on interpretable machine learning with an application to health care demand
مقال من تأليف: Gao, Jieying ; Ye, Tao ; Chu, Dongxiao ; Xu, Xin ;
ملخص: The zero-inflated count data model has long been viewed as an important research topic owing to its enormously different disciplines. As early classical statistical models of linear and logarithmic mean transformation are difficult to be consistent with reality, an enhanced hurdle model based on machine learning methods is proposed. The decision tree, random forest, support vector, and XGBoost methods are introduced in the two stages of the hurdle model. This framework allows to capture the decision-making behavior and predict the count more flexibly and accurately. The generalized hurdle model consists of traditional discrete distributions, which can fit under-dispersed, equi-dispersed, or over-dispersed count data. The extended hurdle models are utilized to fit health care data and compare their performance with traditional count models. The results show that the generalized hurdle model with random forest performs best. Variable importance, break-down plots, and partial plots provide better interpretability for the extended model, which makes the results more reliable and transparent. To the best of our knowledge, this is the first study to generalize the hurdle model with interpretable machine learning methods in count data.
لغة:
إنجليزية
الفهرس العشري
006.3 .الذكاء الاصطناعي (يعمل بشكل عام على الذكاء الاصطناعي والعلوم المعرفية ، والاعتراف من أشكال كأداة الذكاء الاصطناعي ، أنظمة السؤال والجواب)
الموضوع
الإعلام الآلي
الكلمات الدالة:
Two-Stage
Interpretable machine learning
Zero-inflated
Generalized hurdle
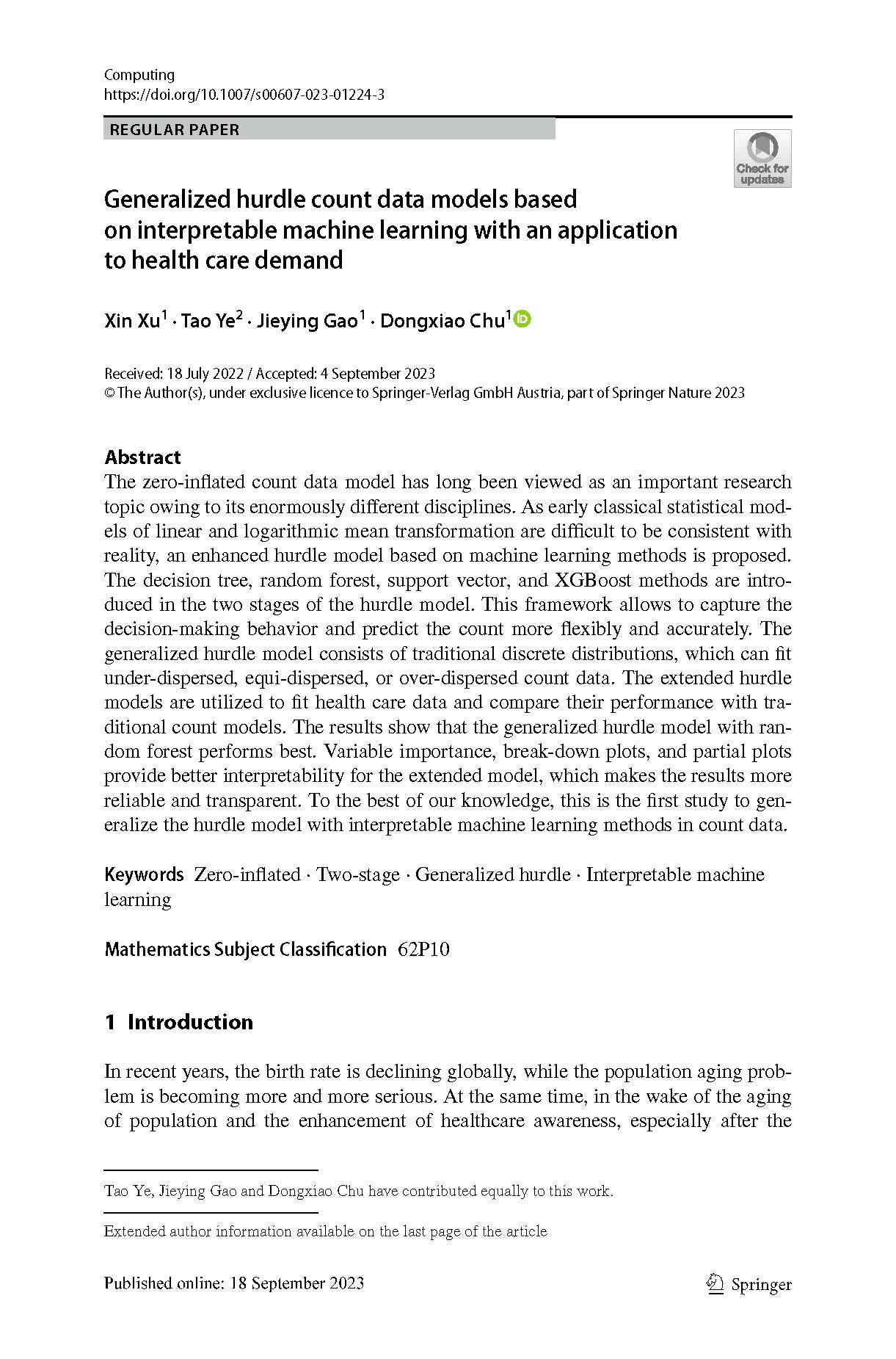