Predicting total sediment load transport in rivers using regression techniques, extreme learning and deep learning models
مقال من تأليف: Kumar, Bimlesh ; Deshpande, Vishal ; Agarwal, Mayank ; Shakya, Deepti ;
ملخص: Total sediment load exerts control over the river channel morphology. Due to the non-linear and multi-dimensional behavior of the variables impacting total sediment load, the prediction is often complex and challenging. To handle this non-linearity and multi-dimensional behavior of total sediment load variables, various approaches like empirical equations, data mining techniques, genetic algorithms, and machine learning (ML) methods have been proposed in the literature. This study provides a substantially better prediction of total sediment load using various methods i.e., linear regression (LR), support vector regression (SVR), extreme learning machine (ELM), and deep neural network (DNN). To evaluate the models, several performance metrics have been used i.e., index of agreement (), Pearson correlation coefficient (PCC), mean square error (MSE), Nash-Sutcliffe efficiency (NSE), root mean square error (RMSE), R, and discrepancy ratio (D). Results show that, the proposed DNN method predicted the total sediment load with significant accuracy and had the highest prediction accuracy (I = 0.989, PCC = 0.979, MSE = 0.042, NSE = 0.958, RMSE = 0.204, R = 0.959, D = 91.91%, respectively) followed by ELM, SVR, and LR. However, the performance of other proposed methods has been satisfactory when compared with the existing techniques. The proposed methods are compared with various well-known empirical equations available in the literature.
لغة:
إنجليزية
الموضوع
الإعلام الآلي
الكلمات الدالة:
Regression analysis
Total sediment load
DNN
ELM
River
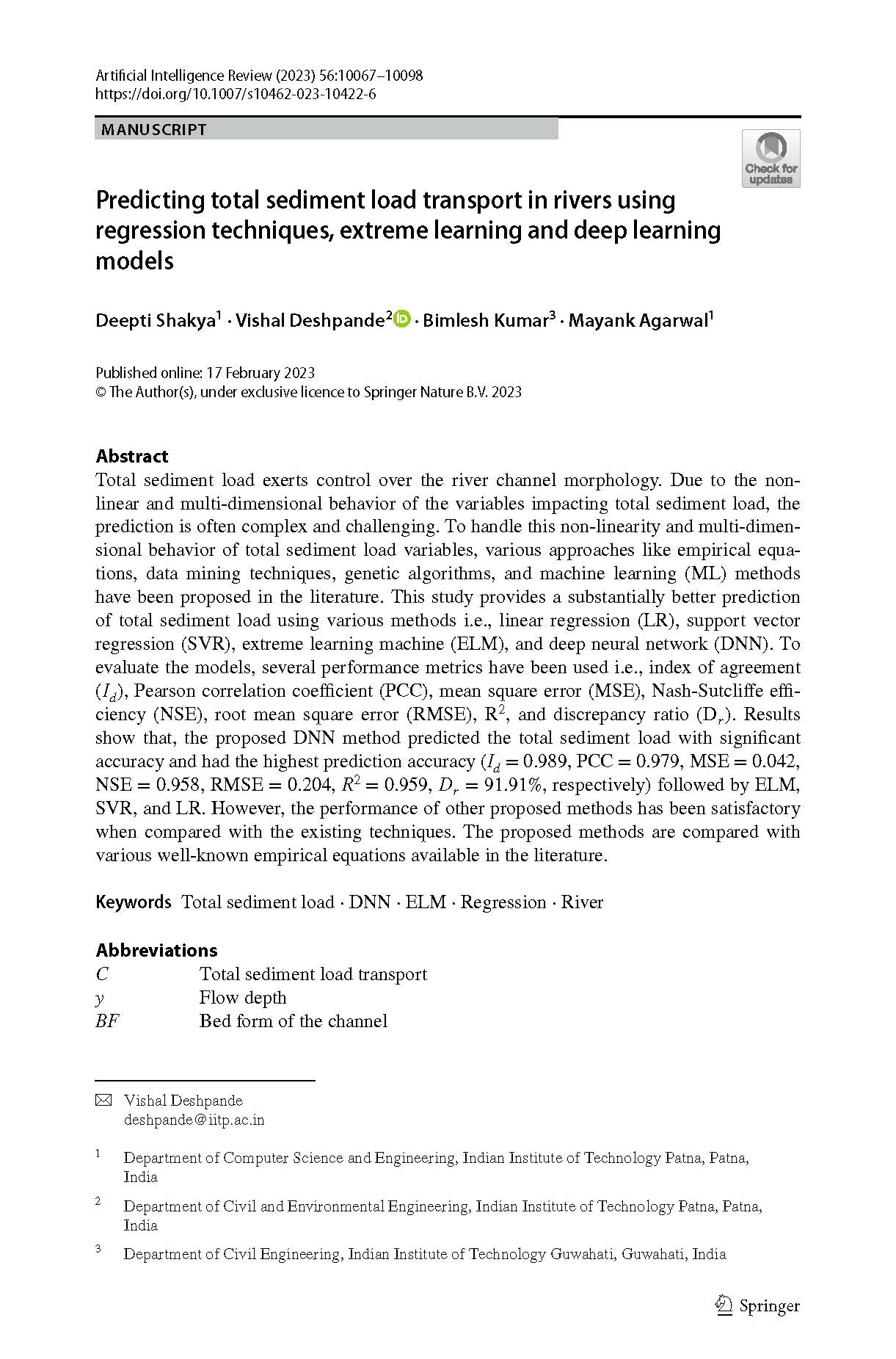