Neural group recommendation based on a probabilistic semantic aggregation
مقال من تأليف: Ortega, Fernando ; Lara-Cabrera, Raul ; Bobadilla, Jesus ; Duenas-Lerin, Jorge ;
ملخص: Recommendation to groups of users is a challenging subfield of recommendation systems. Its key concept is how and where to make the aggregation of each set of user information into an individual entity, such as a ranked recommendation list, a virtual user, or a multi-hot input vector encoding. This paper proposes an innovative strategy where aggregation is made in the multi-hot vector that feeds the neural network model. The aggregation provides a probabilistic semantic, and the resulting input vectors feed a model that is able to conveniently generalize the group recommendation from the individual predictions. Furthermore, using the proposed architecture, group recommendations can be obtained by simply feedforwarding the pre-trained model with individual ratings; that is, without the need to obtain datasets containing group of user information, and without the need of running two separate trainings (individual and group). This approach also avoids maintaining two different models to support both individual and group learning. Experiments have tested the proposed architecture using three representative collaborative filtering datasets and a series of baselines; results show suitable accuracy improvements compared to the state of the art
لغة:
إنجليزية
الموضوع
الإعلام الآلي
الكلمات الدالة:
Collaborative filtering
Deep learning
Group recommender system
Aggregation models
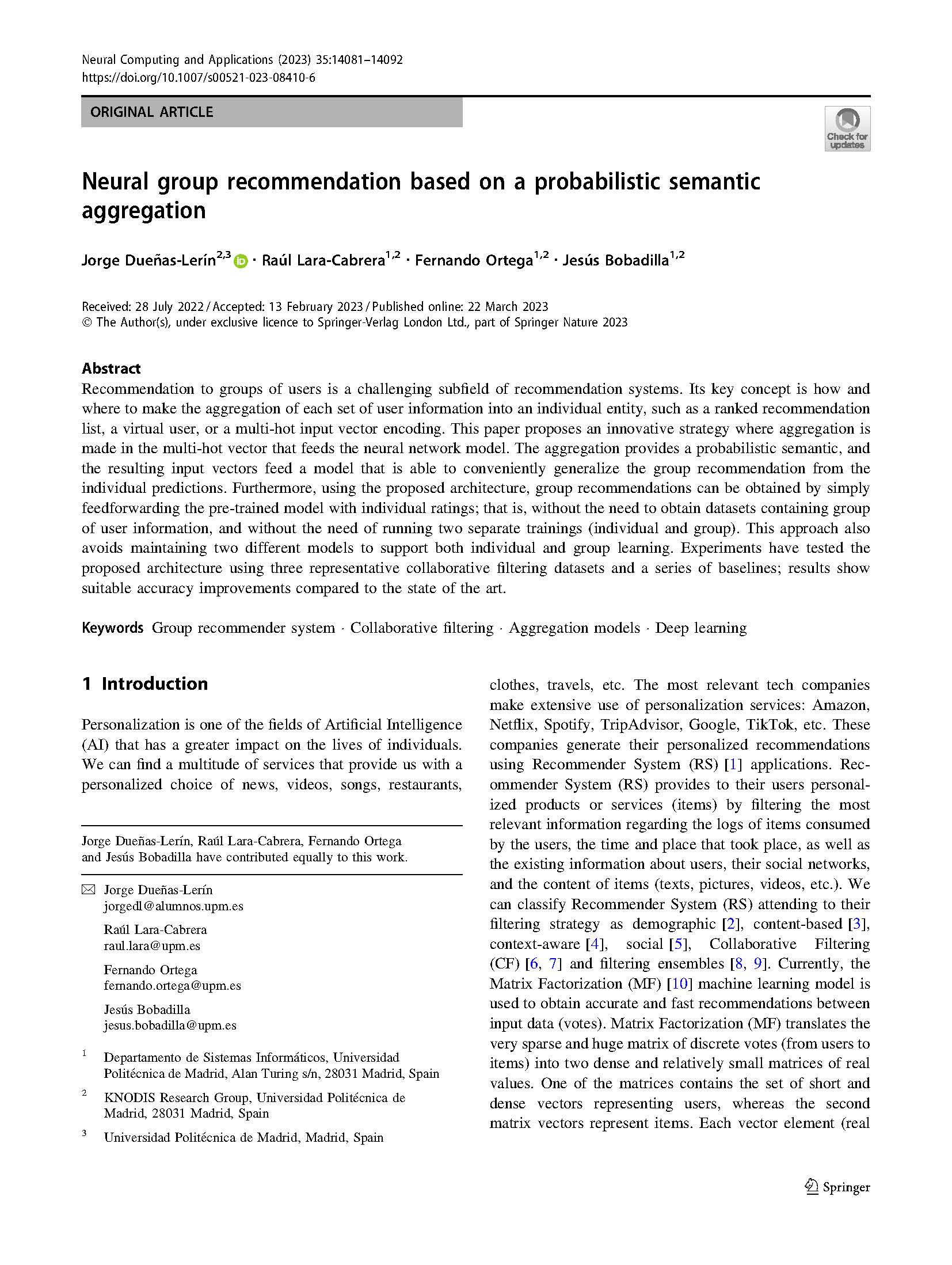