Incremental learning without looking back
a neural connection relocation approach
مقال من تأليف: Zheng, Zejia ; Bo, Yuming ; Wu, Xiang ; Yin, Mingfeng ; Liu, Yi ;
ملخص: Nowadays, artificial intelligence methods need to face more and more open application scenarios. They need to have the ability to continuously develop new skills and knowledge to respond to changes over time. However, how the learning system learns new tasks without affecting performance on old tasks remains a big challenge. In this work, we develop a learning system based on convolutional neural network (CNN) to implement the incremental learning mode for image classification tasks. Inspired by the way human learns, which includes abstracting learning experiences, keeping only key information in mind and forgetting trivial details, our proposed method contains a neural connection relocation mechanism to remove unimportant information from learned memory. And a mechanism composed of knowledge distillation and fine-tuning is also included to consolidate the learned knowledge using associations with the new task. To demonstrate the performance of our method, two pairs of image classification tasks are conducted with different CNN architectures. The experimental results show that our method performs better than the state of the art incremental learning methods.
لغة:
إنجليزية
الموضوع
الإعلام الآلي
الكلمات الدالة:
Distillation
Incremental learning
Convolutional neural network
Neural connection relocation
Filter pruning
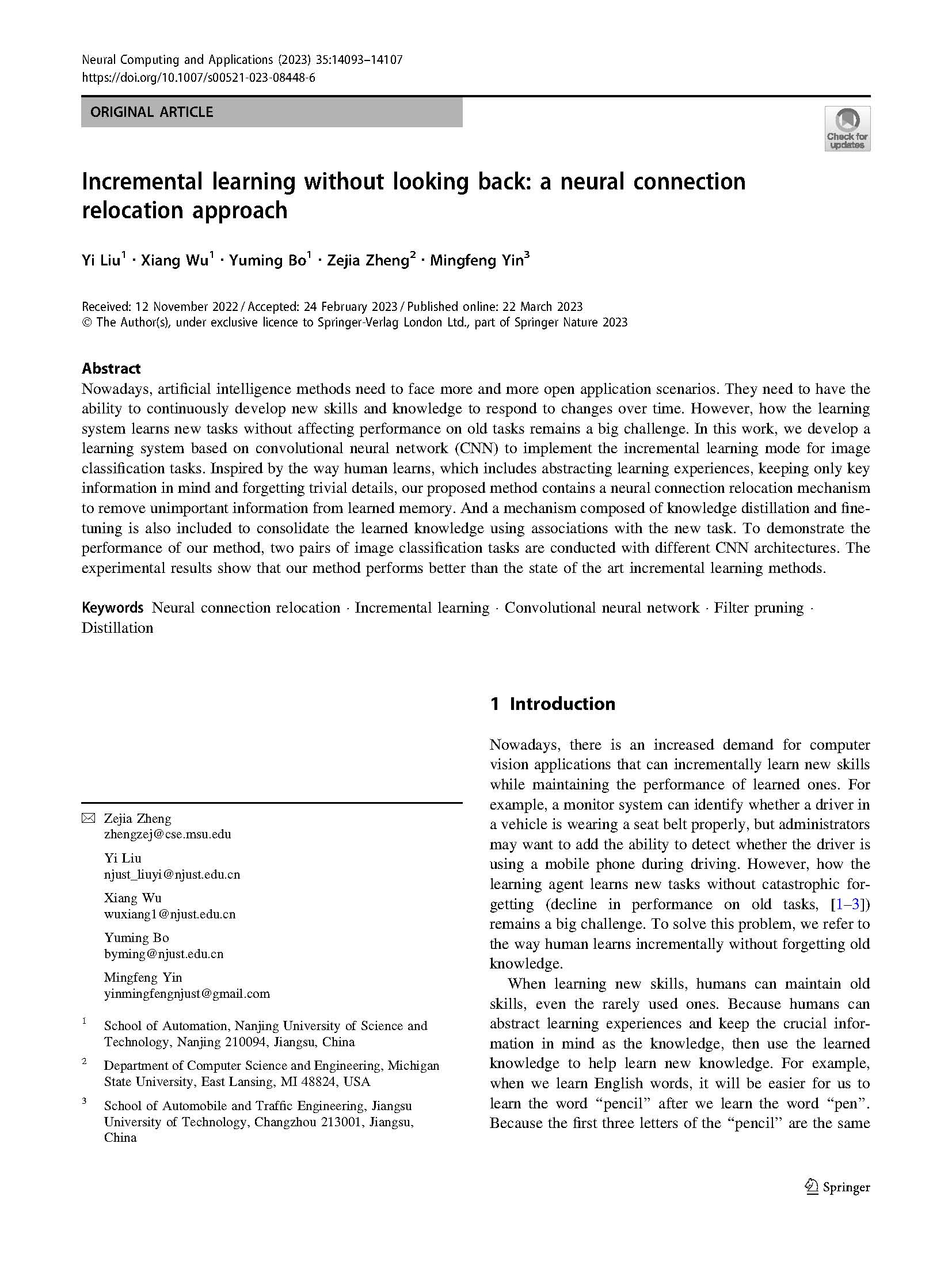