WriterINet
a multi-path deep CNN for offline text-independent writer identification
Article Ecrit par: Ruichek, Y. ; El merabet, Y. ; Touahni, R. ; Chahi, A. ;
Résumé: Handwriting-based identification is a fundamental pattern recognition problem that has attracted considerable interest in recent years. Writer identification is a major challenge, considering how diverse the written content is and how much handwriting differs between writers. This paper presents WriterINet, a CNN-based approach to learning and characterizing each writer's writing style. The proposed WriterINet approach takes handwritten documents as input, decomposing each of them into word images and connected component sub-images. Each segmented image is then fed into our feature learning step to generate discriminative and deep features. To this end, we developed a powerful deep CNN feature architecture consisting of two CNN streams derived from fine-tuning the ResNet-50 and DenseNet-201 models. Deep learned features are computed from all segmented images representing the writer's document and classified using a proposed 1D artificial neural network for predicting writer identification by averaging the similarity scores. Experimental results on IAM, ICDAR2013, CVL, IFNENIT, ICDAR2011, Firemaker and CERUG show that our WriterINet approach achieves the highest or competitive performance over the state-of-the-art.
Langue:
Anglais
Thème
Informatique
Mots clés:
Deep learning
Handwriting
Convolutional neural networks (CNNs)
Feature learning
Text-independent
Off-line writer identification
1D artificial neural network
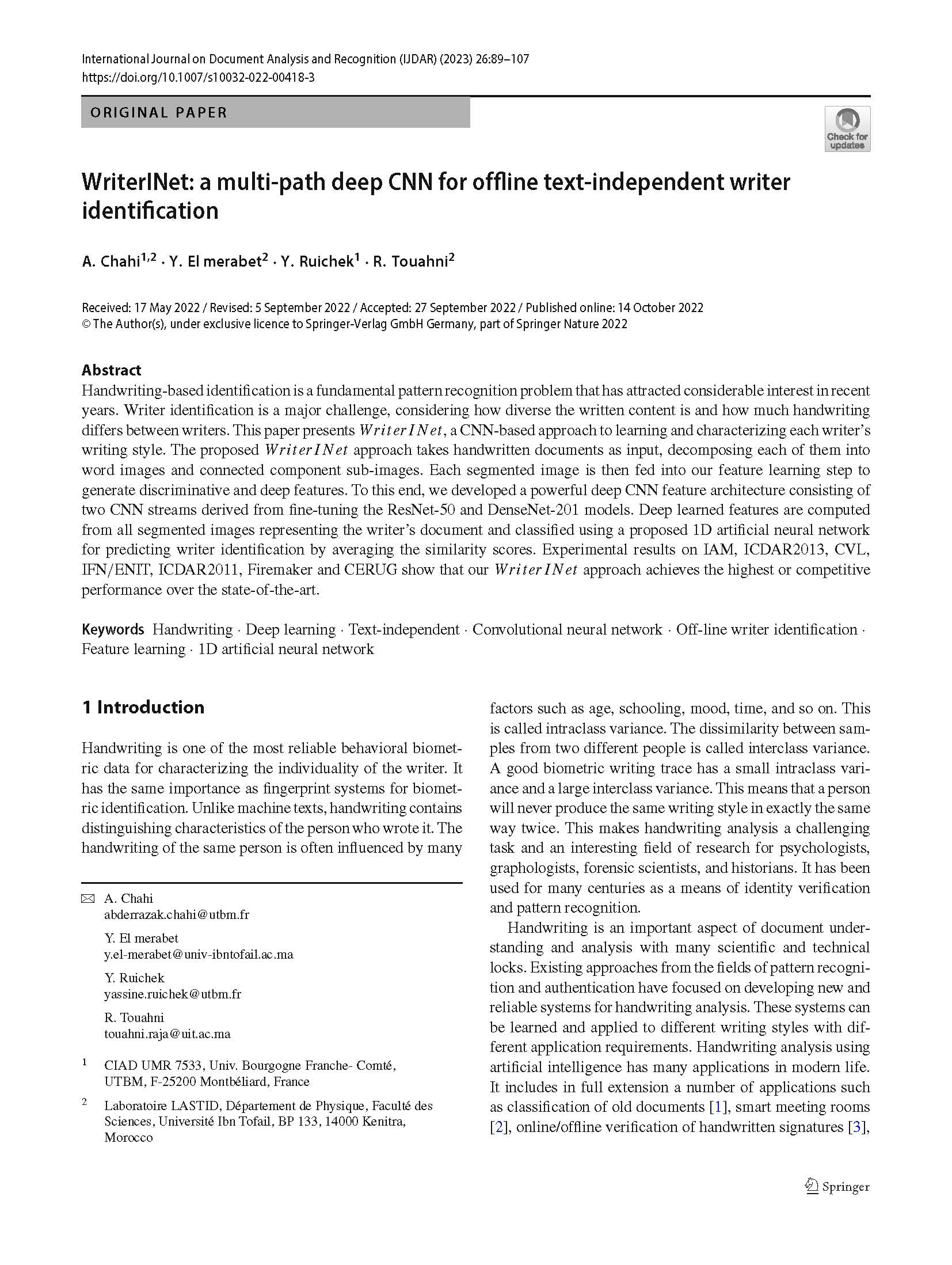