Historical document image analysis using controlled data for pre-training
Article Ecrit par: Ingold, Rolf ; Vogtlin, Lars ; Rahal, Najoua ;
Résumé: Using neural networks for semantic labeling has become a dominant technique for layout analysis of historical document images. However, to train or fine-tune appropriate models, large labeled datasets are needed. This paper addresses the case when only limited labeled data are available and promotes a novel approach using so-called controlled data to pre-train the networks. Two different strategies are proposed: The first addresses the real labeling task by using artificial data; the second uses real data to pre-train the networks with a pretext task. To assess these strategies, a large set of experiments has been carried out on a text line detection and classification task using different variants of U-Net. The observations, obtained from two different datasets, show that globally the approach reduces the training time while offering similar or better performance. Furthermore, the effect is bigger on lightweight network architectures.
Langue:
Anglais
Thème
Informatique
Mots clés:
neural networks
Layout analysis
Historical document images
Training strategies
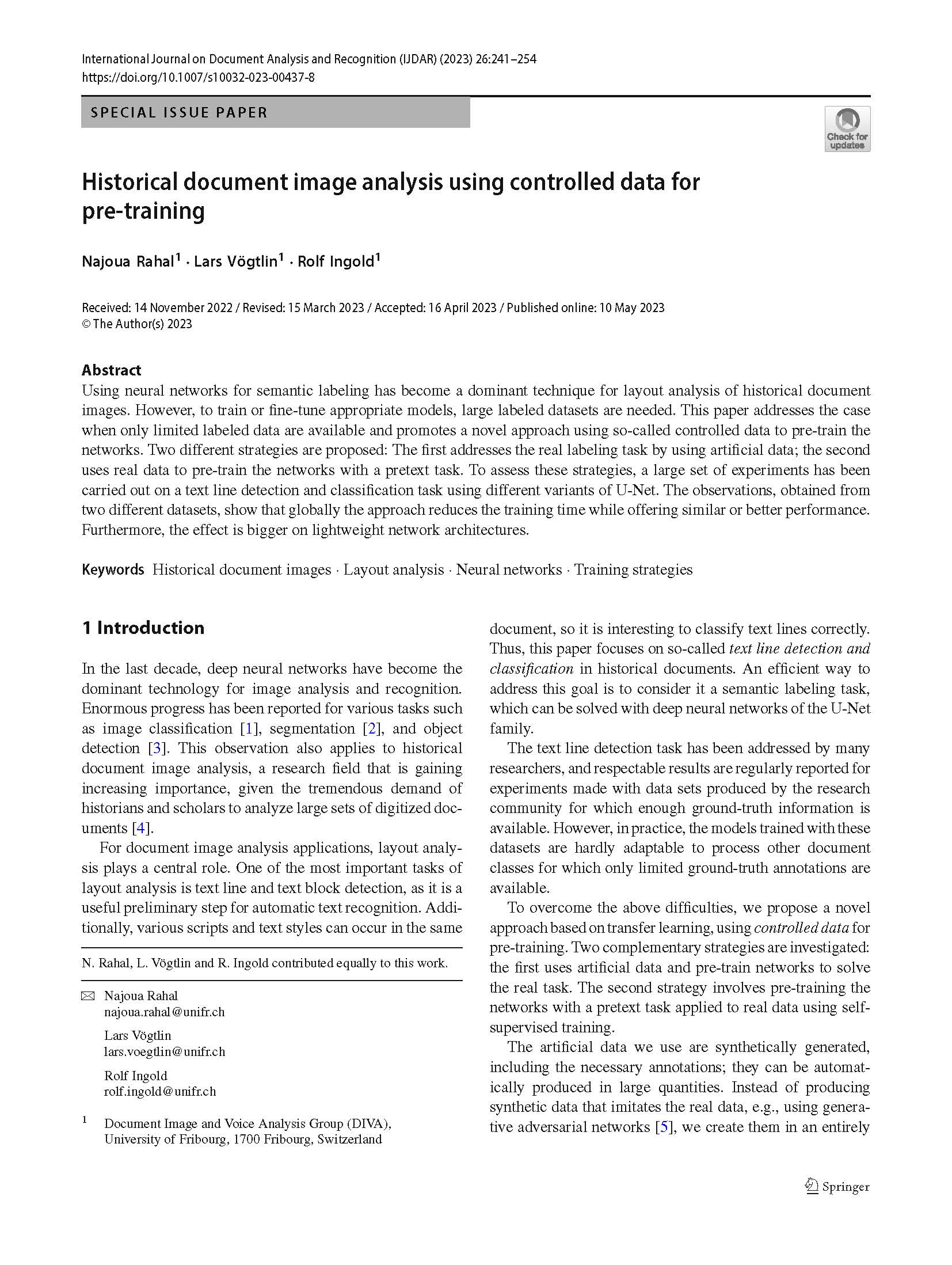