Line extraction in handwritten documents via instance segmentation
Article Ecrit par: Anjum, Tayaba ; Khan, Nazar ; Islam, Adeela ;
Résumé: Extraction of text lines from handwritten document images is important for downstream text recognition tasks. It is challenging since handwritten documents do not follow strict rules. Significant variations in line, word, and character spacing and line skews are acceptable as long as the text remains legible. Traditional rule-based methods that work well for printed documents do not carry over to the handwritten domain. In this work, lines are treated as objects to leverage the power of deep learning-based object detection and segmentation frameworks. A key benefit of learnable models is that lines can be implicitly defined through annotations of training images which allows unwanted textual content to be ignored when required. A deep instance segmentation model trained in end-to-end fashion without any dataset-specific pre- or post-processing achieves 0.858 pixel IU and 0.899 line IU scores averaged over 9 different datasets comprising a wide variety of handwritten scripts, layouts, page backgrounds, line orientations, and interline spacings. It achieves state-of-the-art results on DIVA-HisDB, VML-AHTE, and READ-BAD datasets and almost state-of-the-art results on Digital Peter, ICDAR2015-HTR, ICDAR2017, and Bozen datasets. We also introduce a new, annotated dataset for Urdu script. Our model trained only on Urdu generalizes to multiple other scripts, indicating that it learns a script-invariant representation of text lines.
Langue:
Anglais
Thème
Informatique
Mots clés:
Deep learning
Instance segmentation
Text line extraction
Multiscript
handwritten
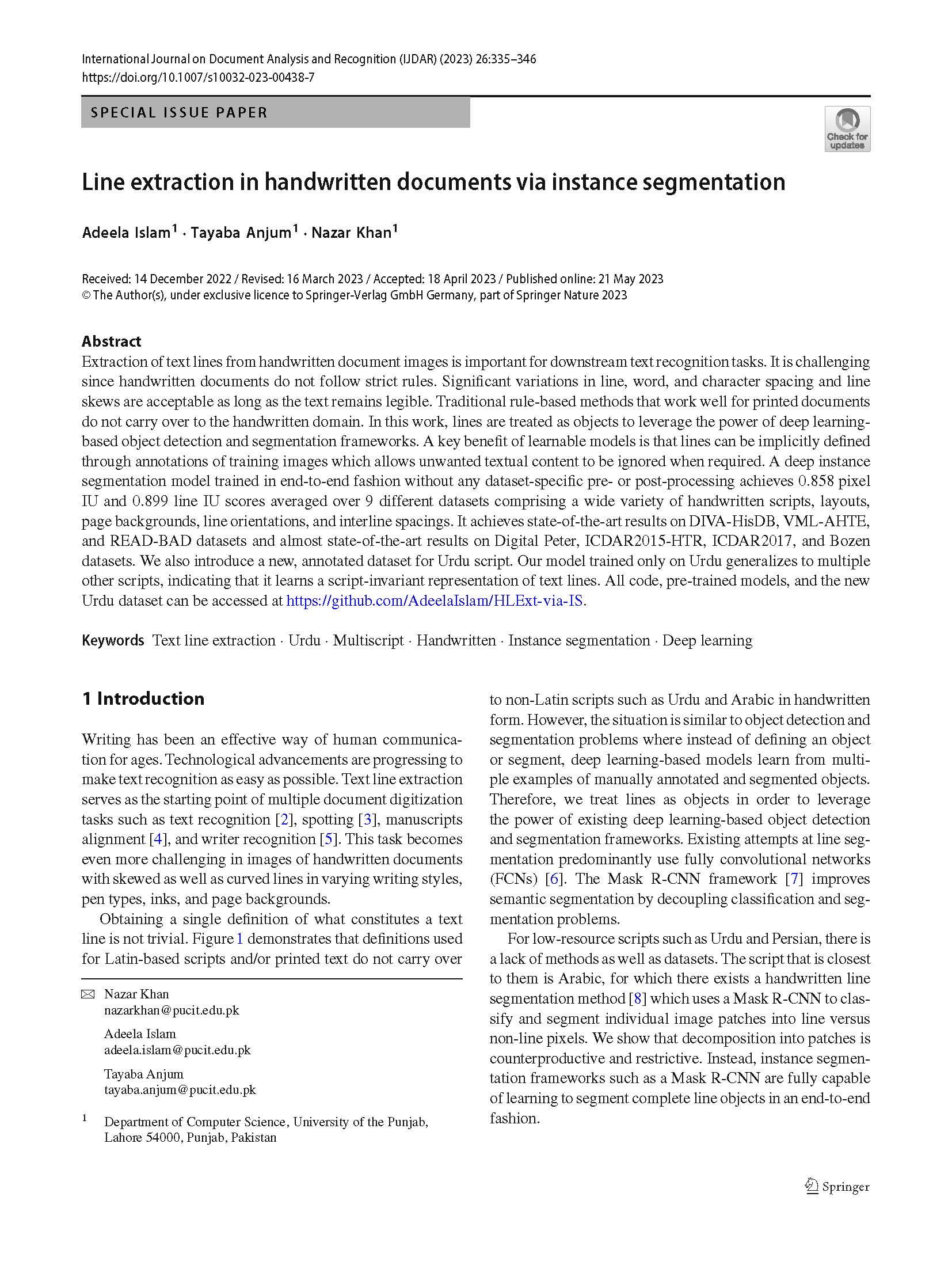