End-to-End Alternating Optimization for Real-World Blind Super Resolution
Article Ecrit par: Wang, Liang ; Tan, Tieniu ; Li, Shang ; Huang, Yan ; Luo, Zhengxiong ;
Résumé: Blind super-resolution (SR) usually involves two sub-problems: (1) estimating the degradation of the given low-resolution (LR) image; (2) super-resolving the LR image to its high-resolution (HR) counterpart. Both problems are ill-posed due to the information loss in the degrading process. Most previous methods try to solve the two problems independently, but often fall into a dilemma: a good super-resolved HR result requires an accurate degradation estimation, which however, is difficult to be obtained without the help of original HR information. To address this issue, instead of considering these two problems independently, we adopt an alternating optimization algorithm, which can estimate the degradation and restore the SR image in a single model. Specifically, we design two convolutional neural modules, namely Restorer and Estimator. Restorer restores the SR image based on the estimated degradation, and Estimator estimates the degradation with the help of the restored SR image. We alternate these two modules repeatedly and unfold this process to form an end-to-end trainable network. In this way, both Restorer and Estimator could get benefited from the intermediate results of each other, and make each sub-problem easier. Moreover, Restorer and Estimator are optimized in an end-to-end manner, thus they could get more tolerant of the estimation deviations of each other and cooperate better to achieve more robust and accurate final results. Extensive experiments on both synthetic datasets and real-world images show that the proposed method can largely outperform state-of-the-art methods and produce more visually favorable results.
Langue:
Anglais
Thème
Informatique
Mots clés:
Blind super resolution
Degradation estimation
Alternating optimization
Restorer
Estimator
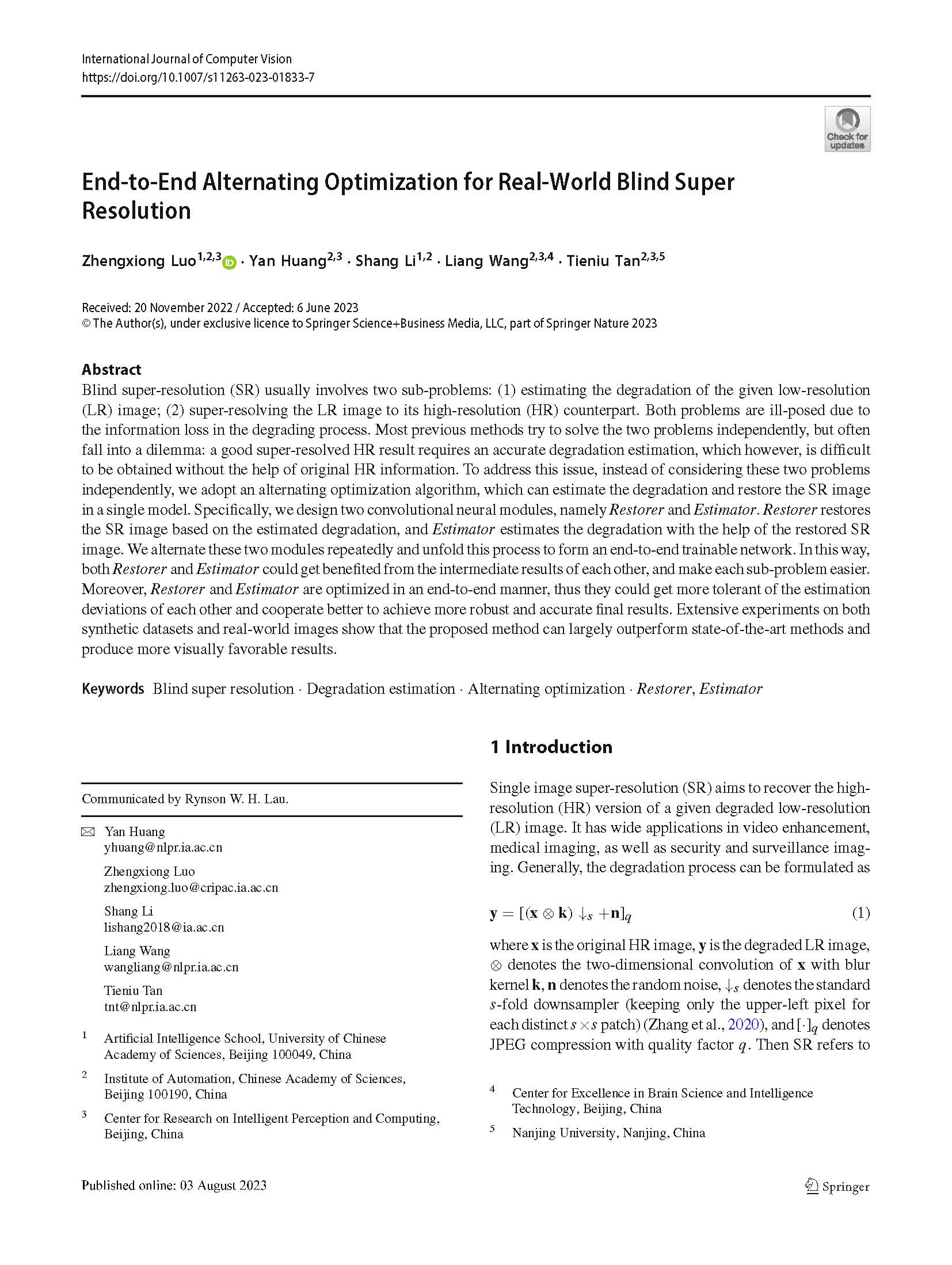