Robots Understanding Contextual Information in Human-Centered Environments Using Weakly Supervised Mask Data Distillation
Article Ecrit par: Nejat, Goldie ; Fung, Angus ; Dworakowski, Daniel ;
Résumé: Contextual information contained within human environments, such as text on signs, symbols and objects provide important information for robots to use for exploration and navigation. To identify and segment contextual information from images obtained in these environments data-driven methods such as Convolutional Neural Networks (CNNs) can be used. However, these methods require significant amounts of human labeled data which is time-consuming to obtain. In this paper, we present the novel Weakly Supervised Mask Data Distillation (WeSuperMaDD) architecture for autonomously generating pseudo segmentation labels (PSLs) using CNNs not specifically trained for the task of text segmentation, e.g., CNNs alternatively trained for: object classification or image captioning. WeSuperMaDD is uniquely able to generate PSLs using learned image features from datasets that are sparse and with limited diversity, which are common in robot navigation tasks in human-centred environments (i.e., malls, stores). Our proposed architecture uses a new mask refinement system which automatically searches for the PSL with the fewest foreground pixels that satisfies cost constraints. This removes the need for handcrafted heuristic rules. Extensive experiments were conducted to validate the performance of WeSuperMaDD in generating PSLs for datasets containing text of various scales, fonts, orientations, curvatures, and perspectives in several indoor/outdoor environments. A detailed comparison study conducted with existing approaches found a significant improvement in PSL quality. Furthermore, an instance segmentation CNN trained using the WeSuperMaDD architecture achieved measurable improvements in accuracy when compared to an instance segmentation CNN trained with Naïve PSLs. We also found our method to have comparable performance to existing text detection methods.
Langue:
Anglais
Thème
Informatique
Mots clés:
Weakly supervised learning for robots
Environment context identification
Segmentation and labeling
Robot navigation and exploration
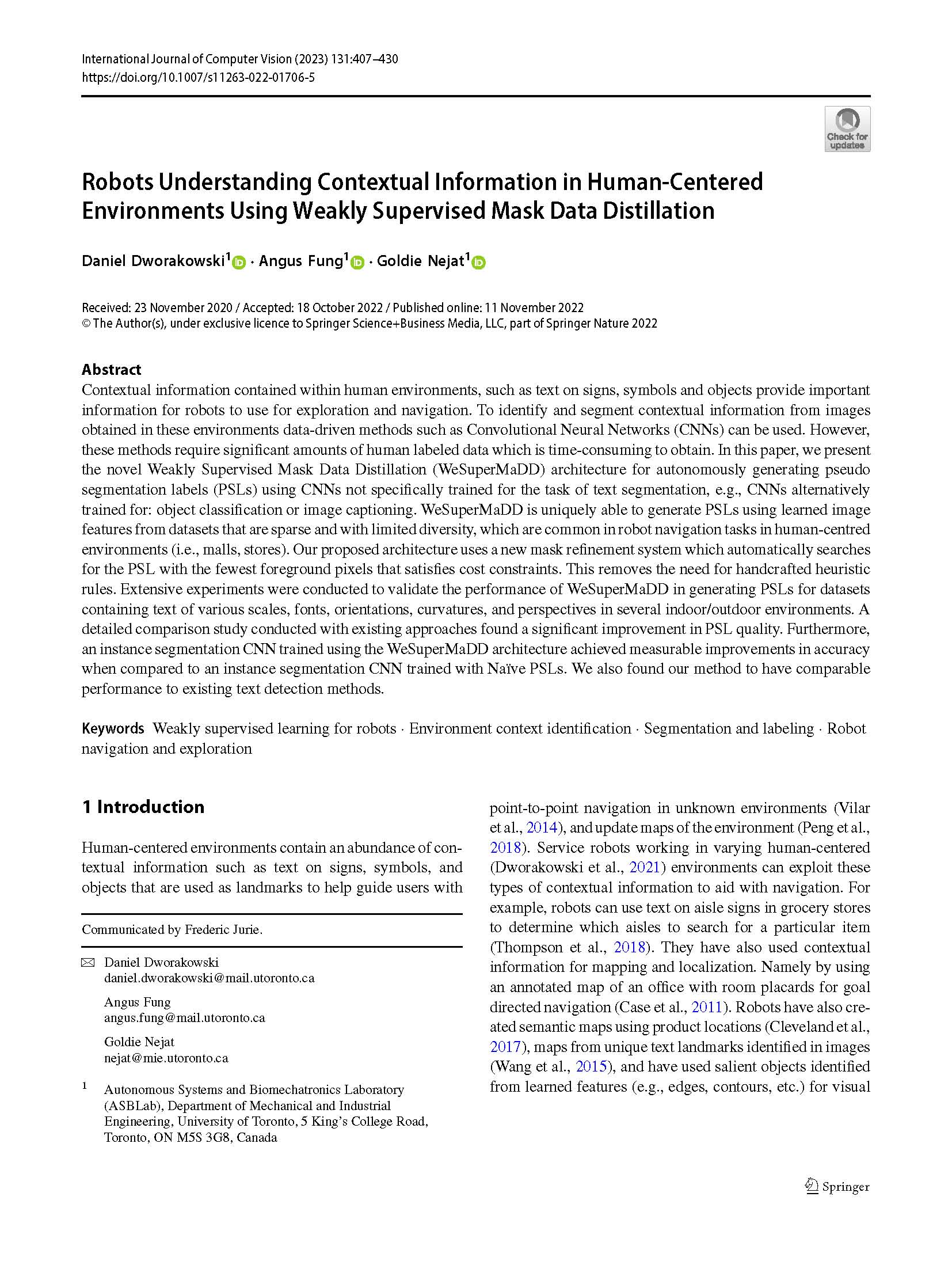