Evaluating SIAMESE Architecture Neural Models for Arabic Textual Similarity and Plagiarism Detection
Article Ecrit par: Aliane, Hassina ; Aliane, Ahmed Amine ;
Résumé: Semantic text similarity in NLP is the study of the degree of resemblance between texts using a certain metric. It has many applications in tasks such as question answering, information retrieval, document clustering, topic detection, topic tracking, questions generation, machine translation, text summarizing and others. Nowadays, neural models are outperforming existing state of the art approaches in major NLP tasks and it is not surprising to see the STS community researchers adopt these models although there are still few works for Arabic language. As Siamese neural architecture has proven recently its relevance for STS in other languages, we evaluate in this work three models within this architecture for Arabic Textual similarity and plagiarism detection: BiLSTM and CNN which we call basic models and a BERT Transformer model.
Langue:
Anglais
Thème
Informatique
Mots clés:
Information retrieval
Measurement error
Knowledge Discovery
semantics
Bit error rate
Buildings
Plagiarism
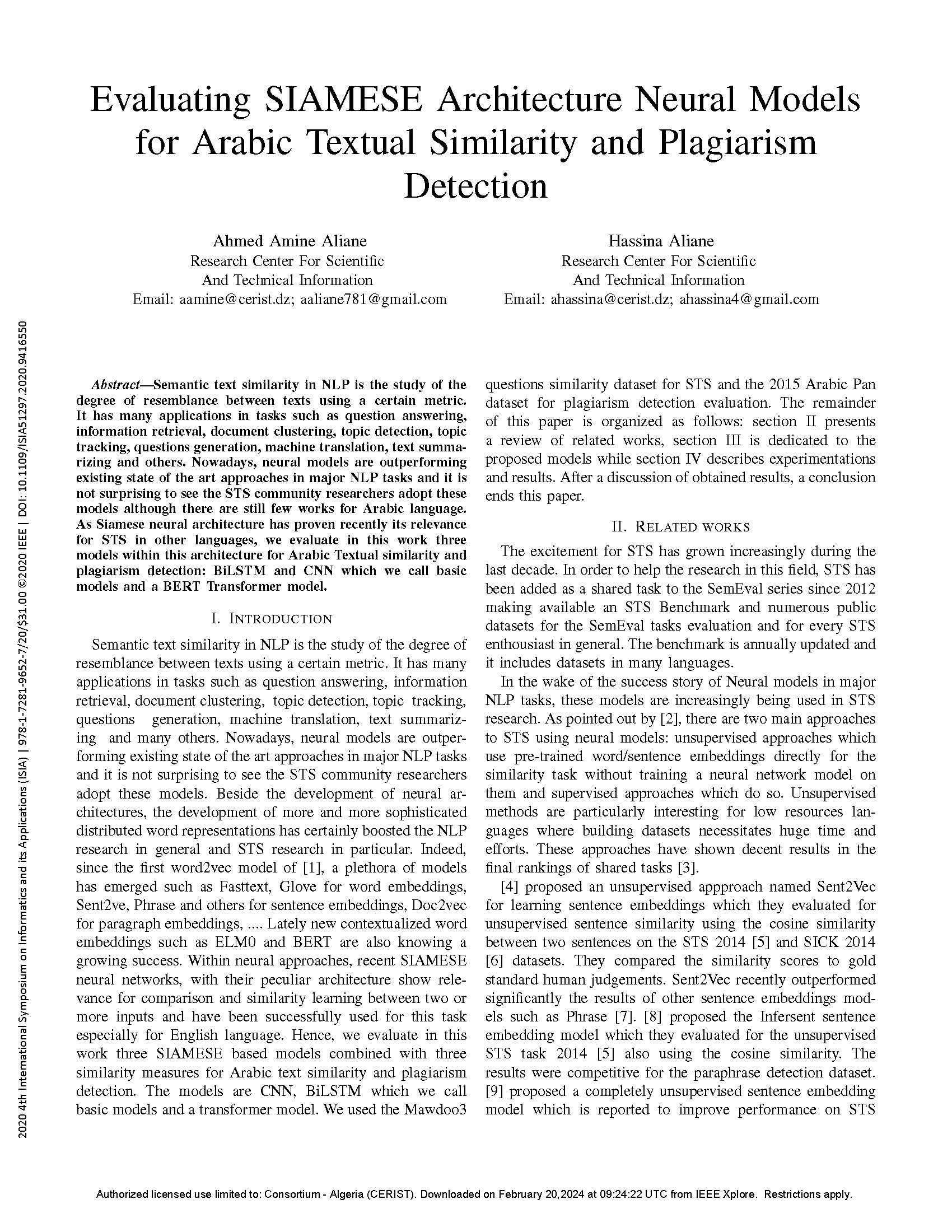