Bayesian Convolutional Neural Networks for Image Classification with Uncertainty Estimation
Article Ecrit par: Laouti, Rayan Y. ; Ghaffar, Maya N. ; Bessai-Mechmache, Fatma Z. ;
Résumé: Over the past decade, deep learning has led to a cutting-edge performance in a variety of fields. However, it faces a fundamental constraint which is the treatment of uncertainty. The representation of the model's uncertainty is of significant importance in areas subject to strict safety or reliability re-quirements. Bayesian deep learning offers a new approach that showcases the degree of reliability of predictions made by neural networks. The present work tests deep learning with Bayesian thinking through a case study of image classification. It puts into practice Bayesian inference to tackle the problem of uncertainty in deep learning and shows its correlation with data quality and model accuracy. To reach this goal, we have implemented a Bayesian convolutional neural network using the variational inference algorithm, Bayes by Backprop. The proposed model was evaluated on an image classification task, with two benchmark datasets. The results' review allowed for validation of the Bayesian approach and showed that it obtains comparable results to those of a non-Bayesian convolutional neural network. Furthermore, the uncertainty of the model was estimated in terms of aleatory and epistemic uncertainty.
Langue:
Anglais
Thème
Informatique
Mots clés:
Image Classification
Convolutional neural networks (CNNs)
Bayesian deep learning
Uncertainty estimation
Bayes by backprop
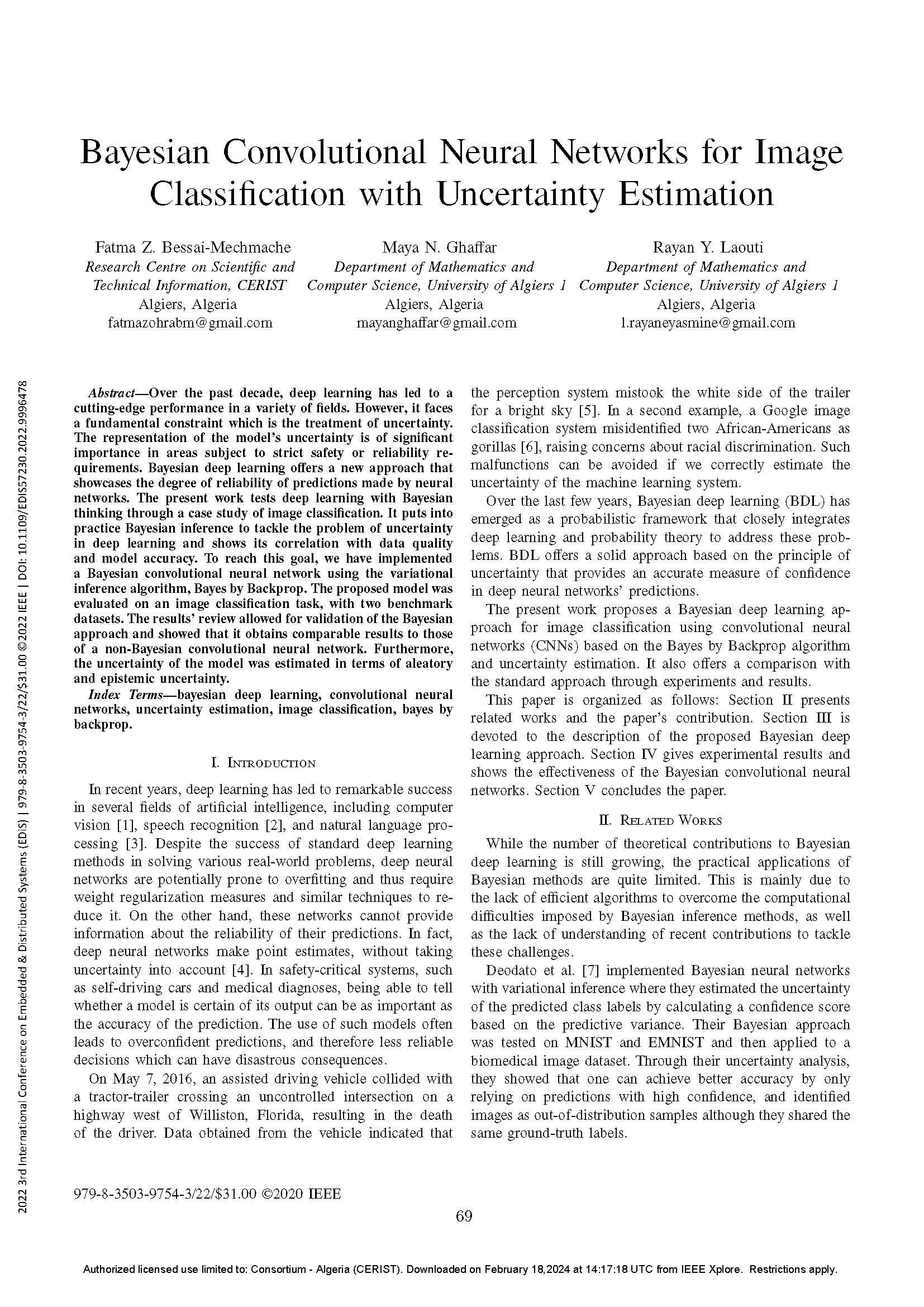